Amazon AIF-C01 Exam is an opportunity for the future!
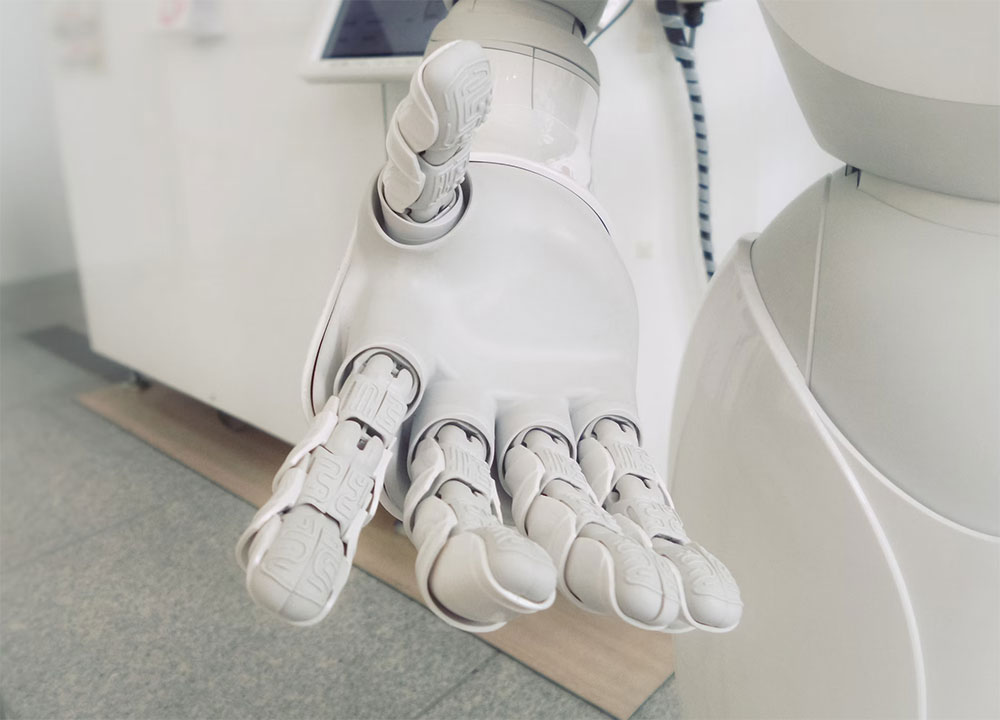
Judging from current data, the global artificial intelligence market is worth more than US$196 billion. In the next six years, the value of the artificial intelligence industry is expected to increase more than 13 times. By 2025, there will be as many as 97 million people working in the field of artificial intelligence. See more Information. So any opportunity to become one of the artificial intelligence workers is very valuable.
CompTIA company summarizes future company AI practitioner data:
22% of companies are actively integrating artificial intelligence into various technology products and business processes.
33% of companies are implementing AI to a limited extent.
45% of companies are still in the exploratory stage.
So if we don’t change, we will eventually be eliminated slowly. This is not alarmist, it is definitely a fact. As one of the world’s largest companies, Amazon’s AI exam certification must be a very popular project. If you If you want to be one of them, keep reading below.
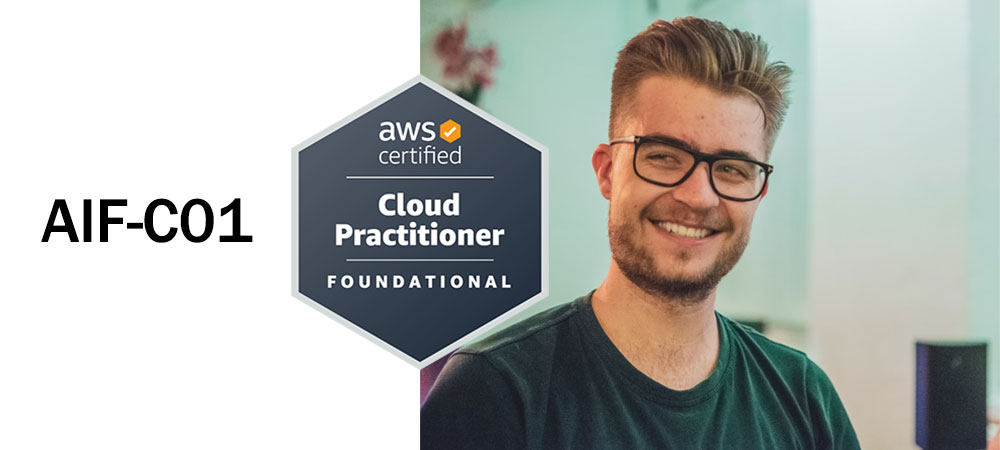
What is the Amazon AIF-C01 exam?
The AWS Certified AI Practitioner (AIF-C01) exam is intended for individuals who can
effectively demonstrate overall knowledge of AI/ML, generative AI technologies, and
associated AWS services and tools, independent of a specific job role.
The exam also validates a candidate’s ability to complete the following tasks:
- Understand AI, ML, and generative AI concepts, methods, and strategies in
general and on AWS. - Understand the appropriate use of AI/ML and generative AI technologies to ask
relevant questions within the candidate’s organization. - Determine the correct types of AI/ML technologies to apply to specific use
cases. - Use AI, ML, and generative AI technologies responsibly.
What is the difference between AWS Certified Machine Learning (MLS-C01)?
First, you must understand that they are different exams and topics, there is no AIF-C01 AI Fundamentals Certificate before 2024. AIF-C01 will not test your project or architecture. AWS Certified Machine Learning (MLS-C01) will. Also, ML != AI, so it depends on the topic you want to show your skills on.
Compared with AWS Certified Machine Learning (MLS-C01) certification, AIF-C01 is a basic certification, which means that the threshold for learning AWS AI solutions is now much lower than before, so for practitioners who want to enter the AI industry Said it was a big opportunity.
Where to get the latest Amazon AIF-C01 exam questions and answers
Here. You can get the latest AIF-C01 exam preparation materials here.
Question 1:
Which option is a use case for generative AI models?
A. Improving network security by using intrusion detection systems
B. Creating photorealistic images from text descriptions for digital marketing
C. Enhancing database performance by using optimized indexing
D. Analyzing financial data to forecast stock market trends
Correct Answer: B
Generative AI models are used to create new content based on existing data. One common use case is generating photorealistic images from text descriptions, which is particularly useful in digital marketing, where visual content is key to engaging potential customers.
Option B (Correct): “Creating photorealistic images from text descriptions for digital marketing”: This is the correct answer because generative AI models, like those offered by Amazon Bedrock, can create images based on text descriptions, making them highly valuable for generating marketing materials.
Option A: “Improving network security by using intrusion detection systems” is incorrect because this is a use case for traditional machine learning models, not generative AI.
Option C: “Enhancing database performance by using optimized indexing” is incorrect as it is unrelated to generative AI.
Option D: “Analyzing financial data to forecast stock market trends” is incorrect because it typically involves predictive modeling rather than generative AI.
AWS AI Practitioner
References:
Use Cases for Generative AI Models on AWS: AWS highlights the use of generative AI for creative content generation, including image creation, text generation, and more, which is suited for digital marketing applications.
Question 2:
A digital devices company wants to predict customer demand for memory hardware. The company does not have coding experience or knowledge of ML algorithms and needs to develop a data-driven predictive model. The company needs to perform analysis on internal data and external data.
Which solution will meet these requirements?
A. Store the data in Amazon S3. Create ML models and demand forecast predictions by using Amazon SageMaker built-in algorithms that use the data from Amazon S3.
B. Import the data into Amazon SageMaker Data Wrangler. Create ML models and demand forecast predictions by using SageMaker built-in algorithms.
C. Import the data into Amazon SageMaker Data Wrangler. Build ML models and demand forecast predictions by using an Amazon Personalize Trending-Now recipe.
D. Import the data into Amazon SageMaker Canvas. Build ML models and demand forecast predictions by selecting the values in the data from SageMaker Canvas.
Correct Answer: D
Amazon SageMaker Canvas is a visual, no-code machine learning interface that allows users to build machine learning models without having any coding experience or knowledge of machine learning algorithms.
It enables users to analyze internal and external data, and make predictions using a guided interface.
Option D (Correct): “Import the data into Amazon SageMaker Canvas. Build ML models and demand forecast predictions by selecting the values in the data from
SageMaker Canvas”: This is the correct answer because SageMaker Canvas is designed for users without coding experience, providing a visual interface to build predictive models with ease.
Option A: “Store the data in Amazon S3 and use SageMaker built-in algorithms” is incorrect because it requires coding knowledge to interact with SageMaker\’s built-in algorithms.
Option B: “Import the data into Amazon SageMaker Data Wrangler” is incorrect. Data Wrangler is primarily for data preparation and not directly focused on creating ML models without coding.
Option C: “Use Amazon Personalize Trending-Now recipe” is incorrect as Amazon Personalize is for building recommendation systems, not for general demand forecasting.
AWS AI Practitioner
References:
Amazon SageMaker Canvas Overview: AWS documentation emphasizes Canvas as a no-code solution for building machine learning models, suitable for business analysts and users with no coding experience.
Question 3:
A company is building an ML model to analyze archived data. The company must perform inference on large datasets that are multiple GBs in size. The company does not need to access the model predictions immediately.
Which Amazon SageMaker inference option will meet these requirements?
A. Batch transform
B. Real-time inference
C. Serverless inference
D. Asynchronous inference
Correct Answer: A
Batch transform in Amazon SageMaker is designed for offline processing of large datasets. It is ideal for scenarios where immediate predictions are not required, and the inference can be done on large datasets that are multiple gigabytes in
size. This method processes data in batches, making it suitable for analyzing archived data without the need for real-time access to predictions.
Option A (Correct): “Batch transform”: This is the correct answer because batch transform is optimized for handling large datasets and is suitable when immediate access to predictions is not required.
Option B: “Real-time inference” is incorrect because it is used for low-latency, real-time prediction needs, which is not required in this case.
Option C: “Serverless inference” is incorrect because it is designed for small-scale, intermittent inference requests, not for large batch processing.
Option D: “Asynchronous inference” is incorrect because it is used when immediate predictions are required, but with high throughput, whereas batch transform is more suitable for very large
datasets.
AWS AI Practitioner
References:
Batch Transform on AWS SageMaker: AWS recommends using batch transform for large datasets when real-time processing is not needed, ensuring cost-effectiveness and scalability.
Question 4:
A company wants to deploy a conversational chatbot to answer customer questions. The chatbot is based on a fine-tuned Amazon SageMaker JumpStart model. The application must comply with multiple regulatory frameworks.
Which capabilities can the company show compliance for? (Select TWO.)
A. Auto scaling inference endpoints
B. Threat detection
C. Data protection
D. Cost optimization
E. Loosely coupled microservices
Correct Answer: BC
To comply with multiple regulatory frameworks, the company must ensure data protection and threat detection. Data protection involves safeguarding sensitive customer information, while threat detection identifies and mitigates security threats to the application.
Option C (Correct): “Data protection”: This is correct because data protection is critical for compliance with privacy and security regulations.
Option B (Correct): “Threat detection”: This is correct because detecting and mitigating threats is essential to maintaining the security posture required for regulatory compliance.
Option A: “Auto-scaling inference endpoints” is incorrect because auto-scaling does not directly relate to regulatory compliance.
Option D: “Cost optimization” is incorrect because it is focused on managing expenses, not compliance.
Option E: “Loosely coupled microservices” is incorrect because this architectural approach does not directly address compliance requirements.
AWS AI Practitioner
References:
AWS Compliance Capabilities: AWS offers services and tools, such as data protection and threat detection, to help companies meet regulatory requirements for security and privacy.
Question 5:
A company wants to classify human genes into 20 categories based on gene characteristics. The company needs an ML algorithm to document how the inner mechanism of the model affects the output.
Which ML algorithm meets these requirements?
A. Decision trees
B. Linear regression
C. Logistic regression
D. Neural networks
Correct Answer: A
Decision trees are an interpretable machine learning algorithm that clearly documents the decision-making process by showing how each input feature affects the output. This transparency is particularly useful when explaining how the model
arrives at a certain decision, making it suitable for classifying genes into categories.
Option A (Correct): “Decision trees”: This is the correct answer because decision trees provide a clear and interpretable representation of how input features influence the model\’s output, making it ideal for understanding the inner mechanisms affecting predictions.
Option B: “Linear regression” is incorrect because it is used for regression tasks, not classification.
Option C: “Logistic regression” is incorrect as it does not provide the same level of interpretability in documenting decision-making processes.
Option D: “Neural networks” is incorrect because they are often considered “black boxes” and do not
easily explain how they arrive at their outputs.
AWS AI Practitioner References:
Interpretable Machine Learning Models on AWS: AWS supports using interpretable models, such as decision trees, for tasks that require clear documentation of how input data affects output decisions.
Question 6:
A loan company is building a generative AI-based solution to offer new applicants discounts based on specific business criteria. The company wants to build and use an AI model responsibly to minimize bias that could negatively affect some customers. Which actions should the company take to meet these requirements? (Select TWO.)
A. Detect imbalances or disparities in the data.
B. Ensure that the model runs frequently.
C. Evaluate the model\’s behavior so that the company can provide transparency to stakeholders.
D. Use the Recall-Oriented Understudy for Gisting Evaluation (ROUGE) technique to ensure that the model is 100% accurate.
E. Ensure that the model\’s inference time is within the accepted limits.
Correct Answer: AC
To build an AI model responsibly and minimize bias, it is essential to ensure fairness and transparency throughout the model development and deployment process. This involves detecting and mitigating data imbalances and thoroughly evaluating the model\’s behavior to understand its impact on different groups.
Option A (Correct): “Detect imbalances or disparities in the data”: This is correct because identifying and addressing data imbalances or disparities is a critical step in reducing bias. AWS provides tools like Amazon SageMaker Clarify to detect bias during data preprocessing and model training.
Option C (Correct): “Evaluate the model\’s behavior so that the company can provide transparency to stakeholders”: This is correct because evaluating the model\’s behavior for fairness and accuracy is key to ensuring that stakeholders understand how the model makes decisions. Transparency is a crucial aspect of responsible AI.
Option B: “Ensure that the model runs frequently” is incorrect because the frequency of model runs does not address bias.
Option D: “Use the Recall-Oriented Understudy for Gisting Evaluation (ROUGE) technique to ensure that the model is 100% accurate” is incorrect because ROUGE is a metric for evaluating the quality of text summarization models, not for minimizing bias.
Option E: “Ensure that the model\’s inference time is within the accepted limits” is incorrect as it relates to performance, not bias reduction.
AWS AI Practitioner References:
Amazon SageMaker Clarify: AWS offers tools such as SageMaker Clarify for detecting bias in datasets and models, and for understanding model behavior to ensure fairness and transparency.
Responsible AI Practices: AWS promotes responsible AI by advocating for fairness, transparency, and inclusivity in model development and deployment.
Question 7:
A company uses Amazon SageMaker for its ML pipeline in a production environment. The company has large input data sizes up to 1 GB and processing times up to 1 hour. The company needs near real-time latency.
Which SageMaker inference option meets these requirements?
A. Real-time inference
B. Serverless inference
C. Asynchronous inference
D. Batch transform
Correct Answer: A
Real-time inference is designed to provide immediate, low-latency predictions, which is necessary when the company requires near real-time latency for its ML models. This option is optimal when there is a need for fast responses, even with Large input data sizes and substantial processing times.
Option A (Correct): “Real-time inference”: This is the correct answer because it supports low-latency requirements, which are essential for real-time applications where quick response times are needed.
Option B: “Serverless inference” is incorrect because it is more suited for intermittent, small-scale inference workloads, not for continuous, large-scale, low-latency needs.
Option C: “Asynchronous inference” is incorrect because it is used for workloads that do not require immediate responses.
Option D: “Batch transform” is incorrect as it is intended for offline, large-batch processing where immediate response is not necessary.
AWS AI Practitioner References:
Amazon SageMaker Inference Options: AWS documentation describes real-time inference as the best solution for applications that require immediate prediction results with low latency.
Question 8:
A company has petabytes of unlabeled customer data to use for an advertisement campaign. The company wants to classify its customers into tiers to advertise and promote the company\’s products.
Which methodology should the company use to meet these requirements?
A. Supervised learning
B. Unsupervised learning
C. Reinforcement learning
D. Reinforcement learning from human feedback (RLHF)
Correct Answer: B
Unsupervised learning is the correct methodology for classifying customers into tiers when the data is unlabeled, as it does not require predefined labels or outputs.
Question 9:
A company wants to use large language models (LLMs) with Amazon Bedrock to develop a chat interface for the company\’s product manuals. The manuals are stored as PDF files.
Which solution meets these requirements MOST cost-effectively?
A. Use prompt engineering to add one PDF file as context to the user prompt when the prompt is submitted to Amazon Bedrock.
B. Use prompt engineering to add all the PDF files as context to the user prompt when the prompt is submitted to Amazon Bedrock.
C. Use all the PDF documents to fine-tune a model with Amazon Bedrock. Use the fine-tuned model to process user prompts.
D. Upload PDF documents to an Amazon Bedrock knowledge base. Use the knowledge base to provide context when users submit prompts to Amazon Bedrock.
Correct Answer: A
Using Amazon Bedrock with large language models (LLMs) allows for efficient utilization of AI to answer queries based on context provided in product manuals. To achieve this cost-effectively, the company should avoid unnecessary use of resources.
Option A (Correct): “Use prompt engineering to add one PDF file as context to the user prompt when the prompt is submitted to Amazon Bedrock”: This is the most cost-effective solution. By using prompt engineering, only the relevant content from one PDF file is added as context to each query. This approach minimizes the amount of data processed, which helps in reducing costs associated with LLMs\’ computational requirements.
Option B: “Use prompt engineering to add all the PDF files as context to the user prompt when the prompt is submitted to Amazon Bedrock” is incorrect. Including all PDF files would increase costs significantly due to the large context size processed by the model.
Option C: “Use all the PDF documents to fine-tune a model with Amazon Bedrock” is incorrect. Fine-tuning a model is more expensive than using prompt engineering, especially if done for multiple documents.
Option D: “Upload PDF documents to an Amazon Bedrock knowledge base” is incorrect because Amazon Bedrock does not have a built-in knowledge base feature for directly managing and querying PDF documents.
AWS AI Practitioner
References:
Prompt Engineering for Cost-Effective AI: AWS emphasizes the importance of using prompt engineering to minimize costs when interacting with LLMs. By carefully selecting relevant context, users can reduce the amount of data processed and save on expenses.
Question 10:
A company is using a pre-trained large language model (LLM) to build a chatbot for product recommendations. The company needs the LLM outputs to be short and written in a specific language.
Which solution will align the LLM response quality with the company\’s expectations?
A. Adjust the prompt.
B. Choose an LLM of a different size.
C. Increase the temperature.
D. Increase the Top K value.
Correct Answer: A
Adjusting the prompt is the correct solution to align the LLM outputs with the company\’s expectations for short, specific language responses.
Question 11:
A large retailer receives thousands of customer support inquiries about products every day. The customer support inquiries need to be processed and responded to quickly. The company wants to implement Agents for Amazon Bedrock.
What are the key benefits of using Amazon Bedrock agents that could help this retailer?
A. Generation of custom foundation models (FMs) to predict customer needs
B. Automation of repetitive tasks and orchestration of complex workflows
C. Automatically calling multiple foundation models (FMs) and consolidating the results
D. Selecting the foundation model (FM) based on predefined criteria and metrics
Correct Answer: B
Amazon Bedrock Agents provide the capability to automate repetitive tasks and orchestrate complex workflows using generative AI models. This is particularly beneficial for customer support inquiries, where quick and efficient processing i crucial.
Option B (Correct): “Automation of repetitive tasks and orchestration of complex workflows”: This is the correct answer because Bedrock Agents can automate common customer service tasks and streamline complex processes, improving response times and efficiency.
Option A: “Generation of custom foundation models (FMs) to predict customer needs” is incorrect as Bedrock agents do not create custom models.
Option C: “Automatically calling multiple foundation models (FMs) and consolidating the results” is incorrect because Bedrock agents focus on task automation rather than combining model outputs.
Option D: “Selecting the foundation model (FM) based on predefined criteria and metrics” is incorrect as Bedrock agents are not designed for selecting models.
AWS AI Practitioner
References:
Amazon Bedrock Documentation: AWS explains that Bedrock Agents automate tasks and manage complex workflows, making them ideal for customer support automation.
Question 12:
Which term describes the numerical representations of real-world objects and concepts that AI and natural language processing (NLP) models use to improve understanding of textual information?
A. Embeddings
B. Tokens
C. Models
D. Binaries
Correct Answer: A
Embeddings are numerical representations of objects (such as words, sentences, or documents) that capture the objects\’ semantic meanings in a form that AI and NLP models can easily understand. These representations help models improve their understanding of textual information by representing concepts in a continuous vector space.
Option A (Correct): “Embeddings”: This is the correct term, as embeddings provide a way for models to learn relationships between different objects in their input space, improving their understanding and processing capabilities.
Option B: “Tokens” are pieces of text used in processing, but they do not capture semantic meanings like embeddings do.
Option C: “Models” are the algorithms that use embeddings and other inputs, not the representations themselves.
Option D: “Binaries” refer to data represented in binary form, which is unrelated to the concept of embeddings.
AWS AI Practitioner References:
Understanding Embeddings in AI and NLP: AWS provides resources and tools, like Amazon SageMaker, that utilize embeddings to represent data in formats suitable for machine learning models.
Question 13:
A student at a university is copying content from generative AI to write essays.
Which challenge of responsible generative AI does this scenario represent?
A. Toxicity
B. Hallucinations
C. Plagiarism
D. Privacy
Correct Answer: C
The scenario where a student copies content from generative AI to write essays represents the challenge ofplagiarismin responsible AI use.
Question 14:
A company has built a solution by using generative AI. The solution uses large language models (LLMs) to translate training manuals from English into other languages. The company wants to evaluate the accuracy of the solution by examining the text generated for the manuals.
Which model evaluation strategy meets these requirements?
A. Bilingual Evaluation Understudy (BLEU)
B. Root mean squared error (RMSE)
C. Recall-Oriented Understudy for Gisting Evaluation (ROUGE)
D. F1 score
Correct Answer: A
BLEU (Bilingual Evaluation Understudy) is a metric used to evaluate the accuracy of machine-generated translations by comparing them against reference translations. It is commonly used for translation tasks to measure how close the generated output is to professional human translations.
Option A (Correct): “Bilingual Evaluation Understudy (BLEU)”: This is the correct answer because BLEU is specifically designed to evaluate the quality of translations, making it suitable for the company\’s use case.
Option B: “Root mean squared error (RMSE)” is incorrect because RMSE is used for regression tasks to measure prediction errors, not translation quality.
Option C: “Recall-Oriented Understudy for Gisting Evaluation (ROUGE)” is incorrect as it is used to evaluate text summarization, not translation.
Option D: “F1 score” is incorrect because it is typically used for classification tasks, not for evaluating translation accuracy.
AWS AI Practitioner
References:
Model Evaluation Metrics on AWS: AWS supports various metrics like BLEU for specific use cases, such as evaluating machine translation models.
Question 15:
How can companies use large language models (LLMs) securely on Amazon Bedrock?
A. Design clear and specific prompts. Configure AWS Identity and Access Management (IAM) roles and policies by using least privilege access.
B. Enable AWS Audit Manager for automatic model evaluation jobs.
C. Enable Amazon Bedrock automatic model evaluation jobs.
D. Use Amazon CloudWatch Logs to make models explainable and to monitor for bias.
Correct Answer: A
To securely use large language models (LLMs) on Amazon Bedrock, companies should design clear and specific prompts to avoid unintended outputs and ensure proper configuration of AWS Identity and Access Management (IAM) roles and policies with the principle of least privilege. This approach limits access to sensitive resources and minimizes the potential impact of security incidents.
Option A (Correct): “Design clear and specific prompts. Configure AWS Identity and Access Management (IAM) roles and policies by using least privilege access”: This is the correct answer as it directly addresses both security practices in prompt design and access management.
Option B: “Enable AWS Audit Manager for automatic model evaluation jobs” is incorrect because Audit Manager is for compliance and auditing, not directly related to secure LLM usage.
Option C: “Enable Amazon Bedrock automatic model evaluation jobs” is incorrect because Bedrock does not provide automatic model evaluation jobs specifically for security purposes.
Option D: “Use Amazon CloudWatch Logs to make models explainable and to monitor for bias” is incorrect because CloudWatch Logs are used for monitoring and not directly for making models explainable or secure.
AWS AI Practitioner References:
Secure AI Practices on AWS: AWS recommends configuring IAM roles and using least privilege access to ensure secure usage of AI models.
…
Best Preparation Materials
geekcert AIF-C01 dumps are the best preparation materials ( https://www.geekcert.com/aif-c01.html ), containing 87 latest exam questions and answers, and are updated in real-time to ensure authenticity and validity, ensuring that you can successfully pass the Amazon AIF-C01 Exam on the first try.
Summarize:
Before taking the Amazon AIF-C01 exam, you must understand all the conceptual knowledge and how to take the exam, and then use the preparation materials provided by geekcert to take effective exam practice tests, which are guaranteed to help you successfully pass the AWS Certified Foundational (AIF-C01) exam.